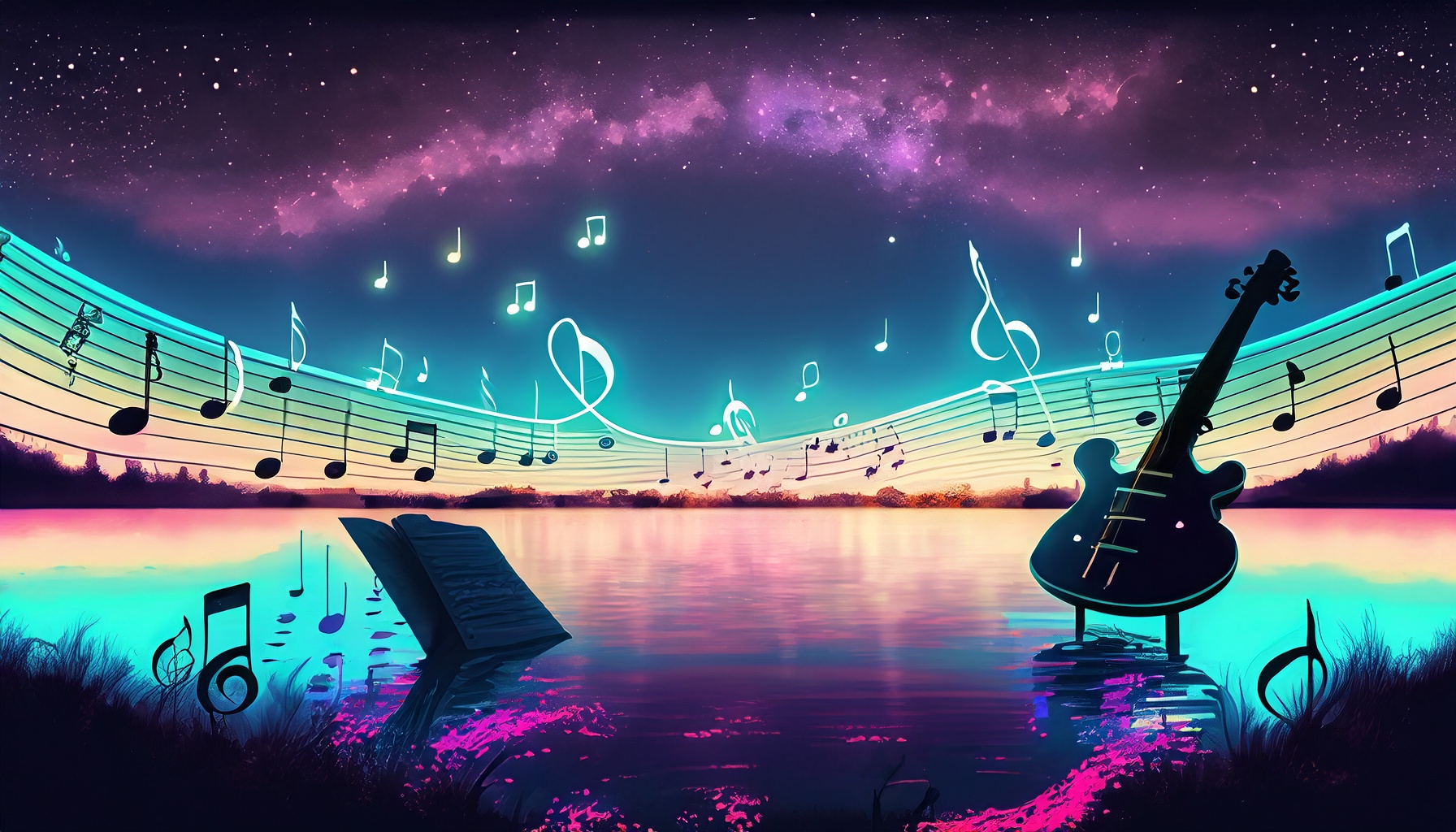
The emergence of generative AI represents a transformative paradigm shift in content creation and interaction, with a specific focus on text and image generation. In recent years, pioneering models such as GPT and DALLĀ·E have achieved significant milestones in the generation of highly realistic images and coherent textual content. These technological advancements have found multifaceted applications across diverse domains, including photorealistic image synthesis, dynamic style transfer, as well as the automated generation of natural language and chatbots. Notably, the stable diffusion models employed for image synthesis have achieved great progress in reducing the computational time while generating high-fidelity images conditioned to the context of the text prompts. This project seeks to explore the novel prospect of employing generative AI models, especially diffusion models, for the purpose of generating acoustic adaptations of musical compositions, referring to the process of generating unamplified, natural-sounding versions of songs, thus contributing to the nascent field of AI-assisted music composition and style transfer.
The main objective of this project is to create a generative AI model specializing in producing acoustic interpretations of musical compositions. Firstly, this project aims to harness the potential of generative AI models to generate quality acoustic renditions of musical compositions in a reasonable amount of time, given the original versions of songs and style requirements during the input stage. Secondly, this project aims to provide a web interface to accompany the model for users to generate musical compositions easily and quickly.
Phase | Time | Milestones | Status |
---|---|---|---|
1 | By 1 October 2023 | Research | Completed |
Detailed project plan | Completed | ||
Project web page | Completed | ||
2 | By late October 2023 | Dataset preparation | Completed |
Audio encoder completed | Completed | ||
By late November 2023 | Text encoder completed | Completed | |
By late December 2023 | Audio splitter completed | Completed | |
By late January 2024 | Audio model completed | Completed | |
Webapp frontend completd | Completed | ||
Webapp backend completed | Completed | ||
3 | By late February 2024 | Models finetuning | Completed |
By late March 2024 | All components completed | Completed | |
By early April 2024 | Testing completed | Completed |